Microsoft has explained how its Quantum team has used AI and high-performance computing (HPC) to quickly identify new materials for battery development in the space of a few days.
Partnering with the Pacific Northwest National Laboratory (PNNL) in Richland, USA, scientists are testing a new battery material that was found using algorithms that reduced 32 million potential inorganic materials to 18 promising candidates that could be used in battery development in just 80 hours.
Microsoft states that this latest project importantly breaks ground for a new way of speeding up solutions for urgent sustainability and other challenges, while giving a glimpse of the advances that will become possible with quantum computing.
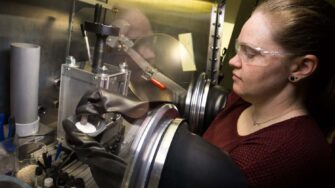
[Image: Dan DeLong for Microsoft]
PNNL is a U.S. Department of Energy laboratory doing research in several areas, including chemistry and materials science, and its objectives include energy security and sustainability.
“The development of novel batteries is an incredibly important global challenge,” said Abrahamson. “It has been a labour-intensive process. Synthesising and testing materials at a human scale is fundamentally limiting.”
Microsoft first trained different AI systems to do sophisticated evaluations of all the workable elements and to suggest combinations. The algorithm proposed 32 million candidates – like finding a needle in a haystack. Next, the AI system found all the materials that were stable. Another AI tool filtered out candidate molecules based on their reactivity, and another based on their potential to conduct energy.
The idea wasn’t to find every single possible needle in the hypothetical haystack, but to find most of the good ones. Microsoft’s AI technology whittled the 32 million candidates down to about 500,000 mostly new stable materials, then down to 800.
“At every step of the simulation where I had to run a quantum chemistry calculation, instead I’m calling the machine learning model. So I still get the insight and the detailed observations that come from running the simulation, but the simulation can be up to half a million times faster,” expained Nathan Baker, Product Leader for Azure Quantum Elements.
Microsoft states that ‘AI may be fast, but it isn’t perfectly accurate’. The next set of filters used HPC, which provides high accuracy but uses a lot of computing power. That makes it a good tool for a smaller set of candidate materials. The first HPC verification used density functional theory to calculate the energy of each material relative to all the other states it could be in. Then came molecular dynamics simulations that combined AI and HPC to analyse the movements of atoms and molecules inside each material.
This process culled the list to 150 candidates. Finally, Microsoft scientists used HPC to evaluate the practicality of each material – availability, cost. etc. – to trim the list to 23 – five of which were already known.
Thanks to this AI-HPC combination, discovering the most promising material candidates took just 80 hours.
The HPC portion accounted for 10 per cent of the time spent computing – and that was on an already-targeted set of molecules.
Microsoft says that the scientists used AI to do the vast majority of the winnowing, accounting for about 90 per cent of the computational time spent. PNNL materials scientists then vetted the short list down to half-a-dozen candidate materials. Microsoft’s AI tools are trained for chemistry, not just battery systems, allowing them to be used for any kind of materials research.