Thangs is an online search tool for 3D, powered by a geometric deep learning engine. We speak to founder Paul Powers about its underlying technology and how it could transform parts procurement
The promise of a ‘Google, but for 3D parts’ has often been made, but little has emerged to live up to the hype. Launched in August, Thangs claims to be different, with the team behind it adamant that its geometric deep learning engine will fill this gap in the market and, in the process, democratise the world of 3D data.
According to parent company Physna, Thangs is able to search for any physical object, including complex assemblies and parts within parts, using text input and 3D file uploads. The cute example we’re given begins with a text search for the name of a Pokemon character: Pikachu.
Thangs quickly gets to work on searching both its own database of over one million models and a plethora of free 3D file sites on the Internet.
Top of the results list is an STL file for Pikachu, found on Thingiverse. As well as several variants, the search also throws up a PokeBall from the same franchise.
“Why?” asks Physna founder and CEO Paul Powers. “It’s not called Pikachu. There’s no Pikachu in the name. There’s no mention of a Pikachu in the metadata.”
The answer, he explains, is that Thangs performs a search based on model geometry alone, using its own proprietary technology to codify the part. This helps it not only find 3D models quickly, but also identify them accurately and understand the relationships between models and similar components.
The same process applies when uploading a 3D model. Say you have the 3D file of a bolt, but you need to know the vendor’s part number or how much it costs. Thangs can search all the available online databases, including those from suppliers such as McMaster-Carr.
In addition to its powerful search capabilities, Powers promises that the Thangs platform is set to grow in its capabilities. Collaboration tools, for example, have already been added to the core technology.
Thangs – searching high and lows
Long-term, Powers wants the wider public to use Thangs to explore the 3D world without needing to learn a CAD software package.
“We took an approach that was purely mathematical and that allowed us to take any kind of file, from any kind of format, and understand every possible relationship – all existing relationships – regardless of whether it’s a part within a part, or a similar part, or a modification of a part, it doesn’t matter,” he explains.
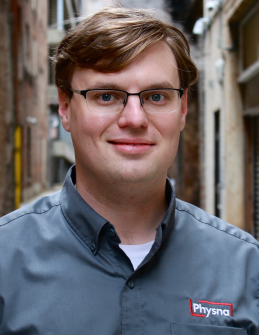
This method lends itself to many other applications. Powers cites use cases like identifying tumours at an earlier stage with 3D artificial intelligence (AI) versus 2D AI, and the automation of mobile phone tower inspection.
“We can’t build all those user interfaces and businesses,” he says, “but we can make Thangs the springboard, where you can use an API to create your own tools using the technology that we’ve developed.”
Powers tells the story of how it all began. By his own description, the “biggest nerd you can imagine”, he travelled from his native US to study law in Germany, where his interest in technology saw him focus on intellectual property (IP) law.
While cases of plagiarism or someone stealing a logo were simple to corroborate, the same didn’t apply to CAD models. There were no algorithms to perform this kind of search and existing online geometric search tools proved no use at all.
“I tried everything that was out there, and nothing worked,” he confirms. The main stumbling block, he explains, was that they couldn’t differentiate between the three-dimensional relationships of parts and assemblies.
With his co-founder, he built the technology for what now forms Physna’s core product offering, able to codify the geometry of nearly 30 different file types and search for them without the need for information on file hierarchy, metadata or BOMs. Thangs, meanwhile, is a free, online offshoot of this core technology.
Physna’s enterprise SaaS offering, with its raft of intuitive features, has been adopted by Fortune 500 companies and US government agencies, many without designers or engineers on their payrolls. It offers amazing benefits for procurement executives, but Powers is adamant that this technology can also help designers and engineers.
Thangs – smarter design
“There are a lot more software inventions than hardware inventions. The reason for that is because of the complexity of inventing something with hardware,” says Powers, explaining that unless it’s a very simple design, components need to be sourced.
“The old adage is that if it takes you two weeks to design something in CAD, it could take you two years to actually source [all the parts],” he says.
What it comes down to, he continues, is identifying, for example, “Who creates that very specific gear that you need, and how do you Google it?”
Powers uses the example of a new watch design uploaded into Physna. The software can show all the components that go inside that watch, and provide information on where the user can source them.
As mentioned, the power of Physna is what powers Thangs, too, with even the free tool offering some neat enterprise-level features. Once logged in, users can create a folder of private models and share it with a team. They can then see how their private models match against everything that’s public, but nobody else can find them. There’s no limit on users or models uploaded.
Another example is of a team of 20 working on building an engine, where the relational database that Thangs builds acts as automated revision control, just like how software designers can pull out, reuse and edit code from GitHub.
“If you’re working on a group project – say, designing an engine, and you’re assigned to design a sections – you’re able to work independently of the rest of the team, but still know how it relates to the greater assembly, because the software has the intelligence to identify the geometry in that workflow and know how the parts relate to one another in an engine assembly,” says Powers.
Naturally, the abilities of Physna go beyond this, into the realm of making predictions for users, based on their habits and behaviours when it comes to cost, manufacturability, volume requirements, materials and so on.
Additional tools take things even further. Powers explains that the Component Finder tool, for example, allows the user to start by designing as little as 1% or even 0.5% of a completed assembly. Once uploaded, Physna will still automatically find parts to build out a complete assembly, all without the need for file hierarchy, file instruction or questioning the 3D CAD system. “Think of it like autofill text,” he laughs.
Physna can help teams speed up the process by not producing duplicates, which in turn, is better for the supply chain. Instead, it can find interchangeable parts, leading to consolidated ordering for things like bolts for different assemblies. That can help to avoid the shutdown of a production line due to a single missing component, a big attraction during the current pandemic, when supply chains are already very vulnerable to disruption.
Finding parts nobody else can
Powers shows us an uncompleted 3D scan of an impeller that clearly went bad. The Scan Match feature in Physna uses the codification of the data to search for completed scans of the same part (including higher resolutions) and, if available, the CAD model for the part.
Because of the way the geometry is codified, you can find CAD with CAD, scans with scans, and CAD with scans. “Any direction you want, which is great,” notes Powers.
“If you pair this with Thangs, you have the ability to scan something and run a search against the public database, to see if the CAD model for the part you’ve just scanned is already out there in the public world.”
Text search, measurements, CAD file and scan data upload can all be used to find whatever it is you are searching for.
Next up? 2D pictures. Powers says it’s coming in the not-too-distant future, claiming that Physna’s proprietary system for 2D-to-3D machine learning puts it way ahead of the likes of Google, Facebook and Adobe in achieving this impressive goal.