Engineers at NASA Goddard Space Flight Center are using generative design to produce lightweight structures for space and taking their AI work on to the next frontier, in the form of text-to-structure workflows, as Stephen Holmes reports
If developing hardware is difficult, then developing hardware for space is on another level. Space agency NASA has more experience in this area than any other organisation worldwide.
“NASA is a cutting-edge organisation and we have an interest in staying on the cutting edge,” smiles Ryan McClelland, speaking from his office at NASA Goddard Space Flight Center.
Located just outside Washington DC, Goddard is a nucleus of design, engineering and science expertise for spaceship, satellite and instrument development, making it a critical ingredient in NASA’s space exploration and scientific missions.
McClelland, a research engineer, is looking to build processes in which AI is used to help design parts and structures, in order to accelerate next-stage space exploration.
Generative design, he says, brings extensive benefits to this work: speed, stronger and lighter parts, and a reduction in the human input necessary. NASA’s total mission cost estimates are in the ballpark of $1 million per kilogram. McClelland shows us an example project, built using generative design, that shaved off 3kg.
“The AI generates the CAD model, generates the design, does the finite element analysis, does the manufacturing simulation – and then you just make it,” he says. “What’s powerful about this is it focuses the engineer on the higherlevel task, which is really thinking deeply about what the design has to do.”
McClelland says this has changed the way his team at NASA Goddard approach design work. “We realised that most engineers take their tools and immediately start designing, sketching and so on without sometimes really fully understanding what the problem is.”
How he and the team now approach a project is by first arriving at a deep understanding of what the part needs to do and then allowing generative design software to build a part that ticks all the boxes. That enables them to get from a list of requirements to machined metal parts in under 48 hours.
Taking giant leaps
Having brought generative design to the fore, the next step was to use AI to start accelerating the process, with text-to-CAD allowing AI to create a form shaped by the list of requirements ran against the large datasets available.
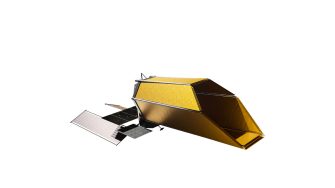
“There’s a real tension between doing things incrementally, so that they’re easy to be absorbed into the workflow, and taking these big leaps, right? So an example of a big leap, is where we’ve used text input,” says McClelland. “It goes through the text-to-structure algorithm and outputs a CAD model, a finite element model and a stress analysis report.”
But from there, the technology can go even further, he explains. “Now we’ve gone and taken that so you can just chat with the AI voice or [use] text, and it creates a JSON file, a standard input file, and then that feeds in. So that’s radically different, right? It skips all the CAD and FEA clicks, and jumps right from, ‘I’m discussing what it is that I want with this agent’, to actually having what I want.”
McClelland explains that while these may indeed be big leaps, they are also laying the groundwork for incremental evolution in people’s workflows.
“In the text-to-structure workflow that I talk about, the underlying technology of generative design-evolved structures is topology optimisation. So, there’s a topology optimisation engine in there that’s like a function call. And ideally, you can just pop that out, right? The Text-to- Structure is somewhat agnostic to what that is. So I think what you’ll see is maybe user interfaces start to fall away.”
He believes that, while today the UIs are a differentiator between CAD softwares, the future will see engineers interface with these systems in a more natural manner.
“[Like] the way that you interface with teams of people, or with people that you want to work with you – so, much more natural interaction, discussing things, sketching things, iterating in a collaborative fashion.”
“We’re basically at the next big paradigm,” he says. “I went and talked to the [NASA] engineer that took us from drawing boards to AutoCAD, and then I talked to the engineer that took us from AutoCAD to what we primarily use now, which is PTC Creo, what used to be ProEngineer. And now we’re ready, I think, for another big step.”
He explains that the majority of the software designers and engineers use today was developed when engineers first had powerful computers sitting on their desk, but before the existence of GPUs, the cloud, and in most cases, the internet.
“I think a lot of those legacy programs have really just taken baby steps to embracing those new compute resources. That next big step is with AI. It really goes along with cloud and GPU and taking advantage of all the amazing things that IT development has to off er the field of engineering.”
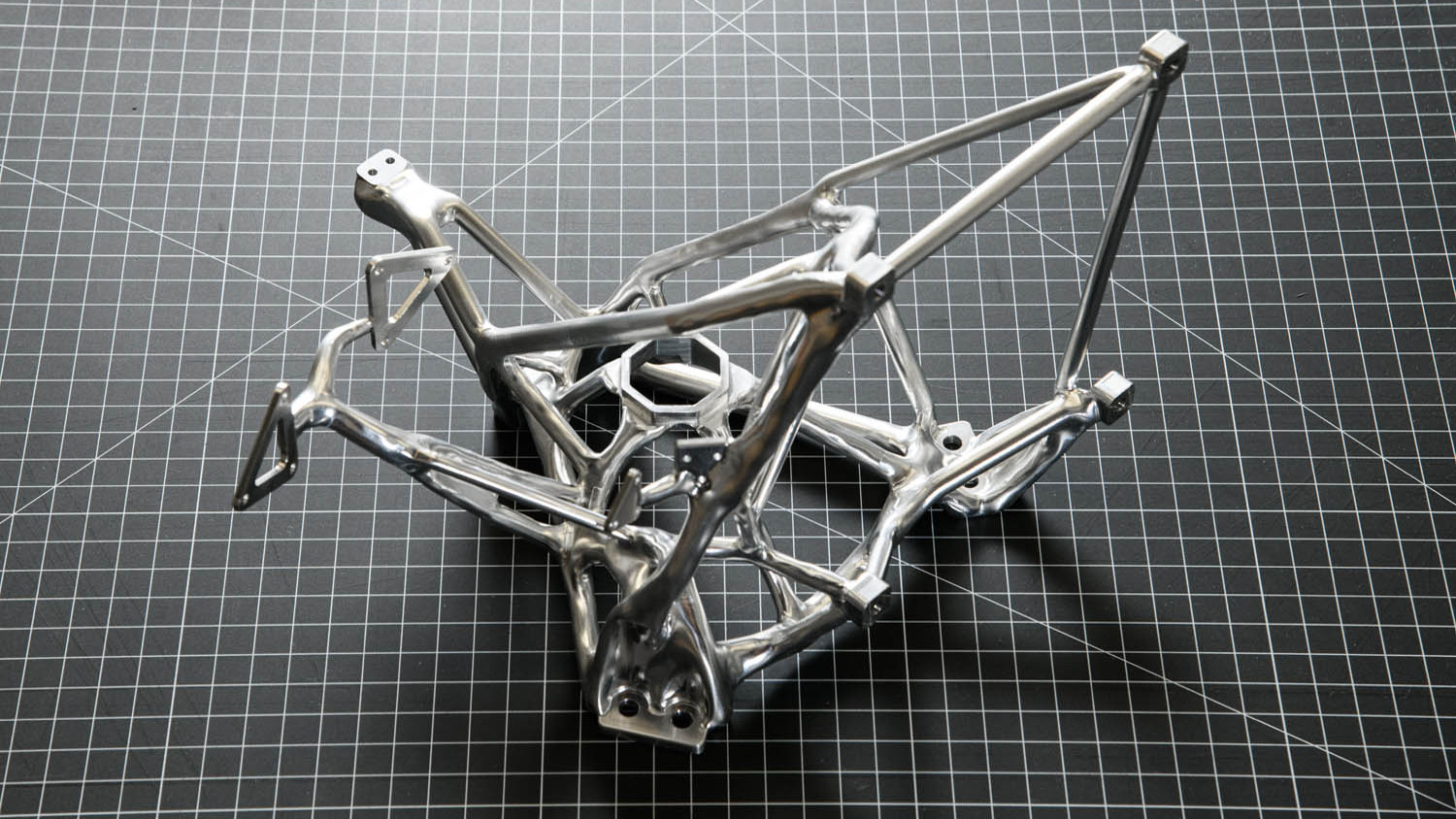
Sprint proposals
During the Covid-19 pandemic, McClelland used the slowdown to take a more in-depth look at what AI might deliver for mechanical engineering. Having been interested in the topic for decades, he began further research into its capabilities. The day ChatGPT went public, he says, was a major milestone in his thinking.
“If you asked my wife, I was wandering around in a daze. I couldn’t believe that we were living in this timeline. The ChatGPT moment brought a lot of new awareness to my work,” he recalls.
Trying out new AI tools at NASA begins with a sprint proposal, a small, 100-hour proposal that can be quickly attained. Having done “a bunch of market research”, McClelland got the green light to try out different AI tools. Once some promise is identified, the next stage focuses on finding applications.
In a facility packed with scientists, engineers and researchers, McClelland began talking to people that might have a need for these AI tools. “I found real applications to apply these to, and that’s how you know if an idea really holds water, when you try it with real needs,” he says.
“We’ve had over 60 applications now, and as you have more applications, you start to find all the edge cases. What are all the complicated factors that you need to take into account that maybe you didn’t think about at first?” To this point, this approach has worked well, resulting in the Evolved Structures Guide.
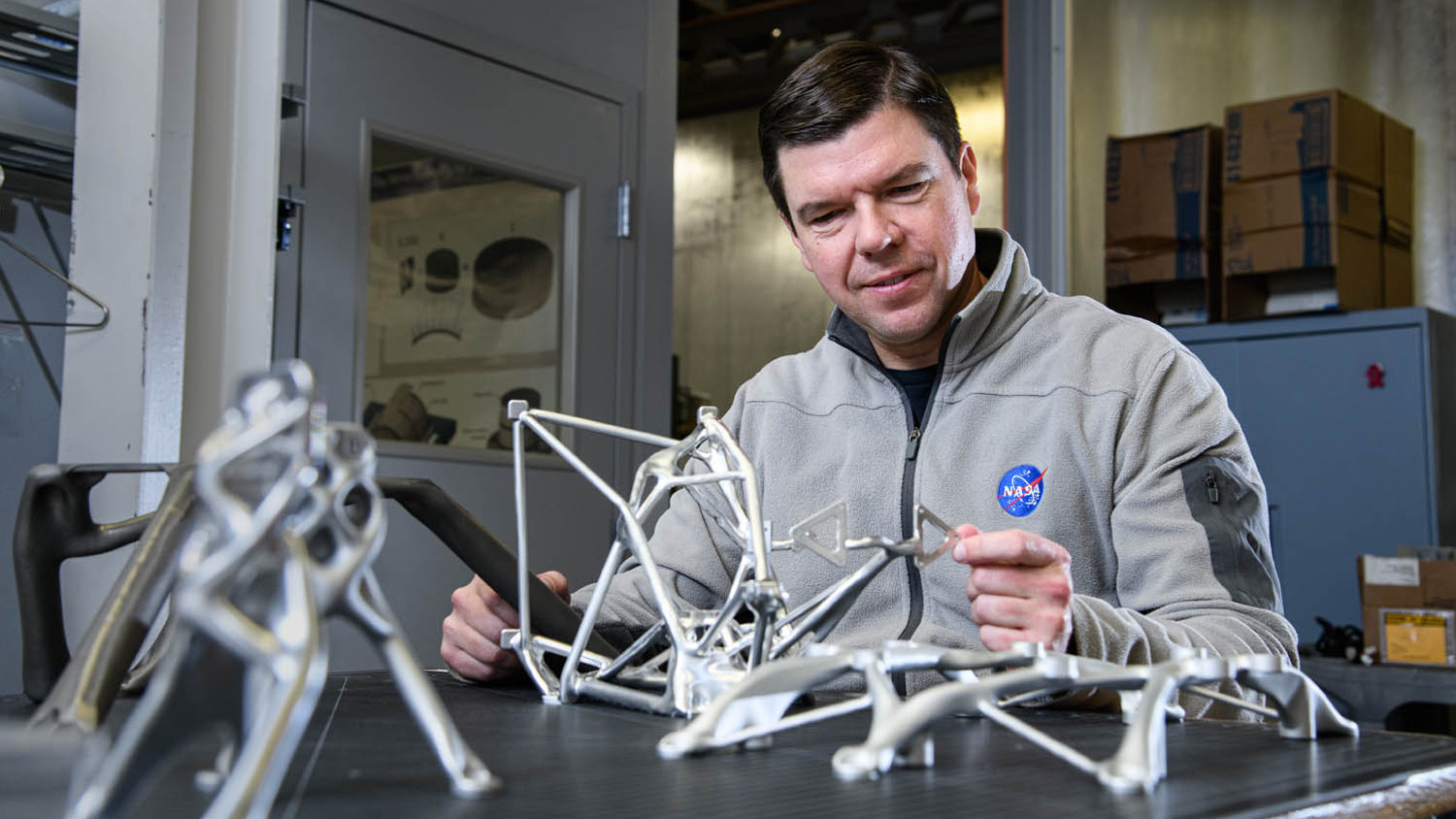
Getting others to adopt this method is challenging, much like getting people to switch to a new CAD software, he says, but can be achieved with training and by explaining the promised benefits.
“You’ve got to demonstrate it. You’ve got to build things. You’ve got to test. That’s what matures the technology, so that it comes into broad usage,” he says.
McClelland says that the full power of AI is, as yet, “under hyped”, and that a lot of people are not factoring its potential into their thinking.
“Fortunately, we have an AI that we can access internally. We have cuttingedge AI tools that we can access with our work data. But I was at a conference recently and I asked people in the audience how many of them can use one of these foundation large language models with their work data with official approval. And, you know, very few people raised their hands.”
Out of this world
With text-to-structure working well, the next stage is to make it more generalisable, says McClelland, so as to cover more of the edge cases. “Which means making it more complex over time, by using real applications on it.”
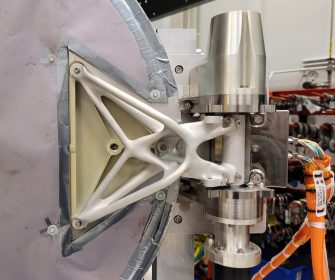
The challenge is lining up the right applications that it can be tested on – the hard bits, he says. “That’s where you find the challenges. Just like how agile software development approaches are used, so that you can get something out there, you have to see what the use cases are, and then iterate, release, iterate.”
A flagship future mission is the Habitable Worlds Observatory, the first telescope designed specifically to search for signs of life on planets orbiting other stars. McClelland hopes that aspects of its design can be accelerated using these AI technologies.
“It’s huge – the size of a three-storey house. You’re going to have these big truss structures, so generating those automatically would be very high-value.”
With no room for error, using AI to generate such structures would prove an incredible benchmark for McClelland and the engineers at Goddard. What it could lead to – and where it might take us next – is entirely out of this world.
This article first appeared in DEVELOP3D Magazine
DEVELOP3D is a publication dedicated to product design + development, from concept to manufacture and the technologies behind it all.
To receive the physical publication or digital issue free, as well as exclusive news and offers, subscribe to DEVELOP3D Magazine here